By Mark Maybury, Chief Technology Officer, Stanley Black & Decker,
23-25 March 2020, AAAI hosted a Virtual Spring Symposium on AI and Manufacturing in which government, academia and commercial participants worked collaboratively to articulate how intelligent manufacturing solutions offer opportunities to improve efficiency, effectiveness and social responsibility. The event chaired by Mark Maybury (Chief Technology Officer, Stanley Black & Decker, [email protected]) was orchestrated by a program committee including Peter Friedland (Science Advisor to AFOSR), Benjamin Gibbs (Co-Founder and CEO, Ready Robotics), Prof. Jim Hender (Director, Rensselaer Polytechnic Institute for Data Exploration and Applications), Prof. John Manferdelli (Executive Director of Northeastern University Center for Cybersecurity and Privacy Institute), and Manish Mehta (Director of Innovation at Stanley Black & Decker). This virtual event featured 18 peer reviewed papers by 57 authors from 22 Institutions along with these three keynotes:
- Doug Maughan, National Science Foundation National Convergence Accelerator, on “The Future of AI and Work”.
- Brad Keywell, President and CEO of Uptake Technologies (and founder of Groupon), on “The Elephant in the AI-enabled Factory: Data Integrity.”
- Marshall Monroe, Conceptualizer of Disney Water Park Blizzard Beach in Walt Disney World, on a robotic cow farm “Old MacDonald Automated His Farm … AI, AI, Oh.”
Motivation
From steam power and electrification in the first industrial revolution to assembly line driven mass production of the second industrial revolution to computerization in the third industrial revolution, disruptive innovations have driven key change including urbanization, global travel, and information discovery and sharing. Equally if not more profoundly, the current cyber-physical fourth industrial transformation is driving fundamental changes not only in the way we manufacture but also because of the kinds of products and services created ways in which we live, work, and play.
Studies from intelligent manufacturing experts at the World Economic Forum have identified a set of key foundational elements for Industry 4.0. These include the Internet of Things (IOT), big data, cloud computing additive manufacturing, augmented reality, autonomous robots, and modeling and simulation. Whether these are deployed individually or integrated into secure solutions, they are assessed collectively to represent a $214B market by 2023 that promises opportunities to achieve $3.7T in value either in revenue generation or cost savings. Increasingly Industry 4.0 products and services leverage artificial intelligence, for example, to automatically perform quality assurance, to anticipate machine failures, to optimize supply chains, or to intelligently physically, cognitively, or communicatively (Maybury 2004) aid humans in task performance.
Since 2014, the industry 4.0 journey has accelerated, empowered by cyber physical systems (Leurent et al. 2018). These systems enable a symbiotic relationship between human and machine intelligence resulting in manufacturing excellence. This acceleration in manufacturing is motivated by factors such as high customer expectations, stressed global supply chains, in a world of accelerating innovation, often driven by AI and machine learning (ML). Organizations that fail to adopt, adapt or augment this intelligent manufacturing trend and compete effectively on the rate of learning and creativity risk extinction.
AI and Manufacturing
Nearly one half million unfilled manufacturing positions (Deloitte 2018), low productivity and strong competition for new products are driving significant need for increasingly intelligent, agile, and collaborative manufacturing. Significant advanced manufacturing activities are underway across the world from global manufactures in a multiplicity of verticals including pharmaceuticals, medical products, automotive, aerospace, consumer goods, construction, power and hand tools, materials, and industrials. Intelligent machines are addressing an increasingly complex range of manufacturing tasks including product design, material handling, machine tending, assembly, packaging, and distribution. Intelligent systems can provide a range of potential benefits such as speed, flexibility, accuracy, and tailorability. Intelligent manufacturing leverage multiple AI disciplines, drawing from (supervised and unsupervised) machine learning, image understanding, spoken language dialogue, media extraction, search, and knowledge representation, among other areas.
AI and Manufacturing 10 Year Roadmap
In preparation work and during interactive symposium sessions, the group refined a 10-year community roadmap for AI in Manufacturing in the near (2020), mid (2025), and far term (2030) as shown in Figure 1. The symposium participants identified three key lanes of progress in data, modeling and analysis, capabilities and applications, and ecoystems with existing technologies depicted in italicized red, offramps of capabilities shown as signposts, and roadblocks in yellow along the way. The first lane depicts the progress in sensors and sources. A massive growth in industrial internet of things (IIOT) is driving challenges in volume, variety, and veracity of data as it progresses from structured (e.g., DBs, tables) to massive, unstructured and uncertain data. Noisy and uncertain data will challenge progress. Existing 3D models of products need to be enriched with adaptive models of the user, process, and domains, with increasingly rich representation and reasoning about spatial, temporal, and causal knowledge. The progress toward deeper models and from predictive to prescriptive analytics will ultimately be enabled by modeling that progresses from current objects and relations to include events and behaviors.
The second lane illustrates advances in capabilities and applications. The plethora of mobile and cooperative robots will be enhanced through advances that overcome roadblocks in vision and dexterity and are increasingly user and context aware, enabling autonomous operations. Existing computer vision algorithms such as simultaneous localization and mapping (SLAM) will be extended with new methods such as multispectral vision in the short term but possibly also quantum sensing and processing in the longer term. This will accelerate vision for the detection of defects, localizing objects in the camera field-of-view, detection of foreign items or object sorting. There will be increasing opportunities for service robots as well as new kinds of robots such as tool using robots (tobots). General lack of trust in AI (e.g., perceived risk or algorithmic bias) will act as a speed limit to progress.
A third and final lane illustrates the measurable progress from useful and usable systems to once that are evolvable and sustainable. More intuitive and collaborative systems that are also increasingly transparent and self-aware will increase the ease of upskilling and reskilling workers with the transformation of the work of the future is transformed to more symbiotic human-machine systems. These advances will accelerate speed, flexibility, and accuracy and support an increasingly complex range of manufacturing tasks including product design, material handling, machine tending, assembly, packaging, and distribution. Intelligent systems will provide a range of potential benefits including eeconomic, operational, and human. Symbiotic systems promise to provide more optimal distribution of tasks among humans and machines … ultimately driving synergistic human-system operations across supply chains, distribution centers and factories. Innovations can play several roles including expert advisor, robotic aid, or expert tutor for upskilling and re-skilling.
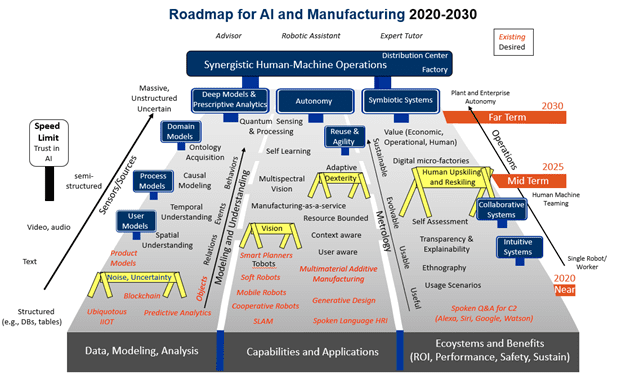
Figure 1. 10 Year Community Roadmap for Intelligent Manufactoring
Participants made contributions in multiple areas of the roadmap, depicted in red in Figure 1, which we summarize herein.
AI for Human Upskilling and Reskilling
Supporting the National Science Foundation Convergence Accelerator, Jennifer Thornton and Stephanie Blockinger from the Business-Higher Education Forum reported on “Empowering a Digital Technology Workforce through Alignment and Coordination of Upskilling and Reskilling Opportunities”. Supported by the National Science Foundation Convergence Accelerator, Huiling Ding (North Carolina State University) discussed approaches for “Building Ethical AI for Workforce Empowerment, Upskilling, and Reemployment in Manufacturing.” Others discussed intelligent training methods. Next, a joint paper by Weichao Wang, Pu Wang and Aidong Lu from the University of North Carolina at Charlotte, Mark Maybury from Stanley Black & Decker and Xintao Wu from the University of Arkansas, described an AI-assisted smart training platform for the future manufacturing workforce. Finally, a team from University of Massachusetts at Amherst including Beverly Woolf, Aritra Ghosh, Andrew Lan, Shlomo Zilberstein and Tom Juravich delivered the paper “AI-Enabled Training in Manufacturing Workforce Development” including not only methods for intelligent tutoring for workers on the manufacturing line but also using AI to mine resumes to provide guidance on manufacturing career paths.
AI to Enhance Sensors and Analytics
Another major theme of the symposium was the application of AI to provide intelligent sensing and analytics. For example, Bijan Sayyarrodsari and Tom O’Reilly from Rockwell Automation reported on real-time analytics for industrial internet of things (IIOT). Ron Stuver, Dave Adams, and Kam Yuen from SICK, Inc. reported on sensors and AI for Factory Automation. Vivek Diwanji, Phani Bhushan Sistu, and Sharath Prasad from Cognizant described AI applications in manufacturing operations.
AI for Generative Design and Additive Manufacturing
Additive manufacturing provides multiple important use cases for AI. Binil Starly, Atin Angrish, Deepak Pahwa, Mahmud Hasan, Akshay Bharadwaj, and Paul Cohen from North Carolina State University described how AI enables the democratization of innovation through design automation in their ‘one-click’ manufacturing services and intelligent machines. Another important design use case is quality. In a joint paper from Vivian Wen Hui Wong, Max Ferguson and Kincho Law from Stanford University and Yung-Tsun Tina Lee and Paul Witherell from the National Institute of Standards and Technology (NIST), the authors report on automatic volumetric segmentation of additive manufacturing defects with 3D U-Net.
AI for Machining, Assembly/Process Control and Optimization
Another valuable area for AI is machining and assembly. Mark Maybury, Sudhi Bangalore, Eric Cohen, Ashley Baron, and Paul Turner from Stanley Black & Decker described several use cases including cooperative robots for machine tending and mobile robots that save up to 20% of worker time in distribution centers by automated material movement. Sastry Malladi from FogHorn described the application of vision and analytics at the edge to reduce scrap in a Stanley Black & Decker manufacturing plant. M-Mahdi and Sadra Naddaf-Sh, and Hassan Zargaradeh from Lamar University together with Mohammad Zahiri and Amir Kashani from Stanley Oil & Gas described an automated weld quality assessment capability using digital radiographic images and deep learning. Noel Greis and Monica Nogueira from North Carolina State University, Sambit Bhattacharya from Fayetteville State University and Tony Schmitz the University of Tennessee Knoxville described their approach to use physics-guided machine learning for self-aware machining. Finally, turning toward predicting natural energy systems, Zhao Li, Yan Tang, and Zequan Guo from Hohai University described their model AccuWave, a stochastic process model for accurate and real-time ocean wave prediction.
Exploration, Annotation, and Assessment
A final session of the symposium was on mobile robotics, training data annotation and assessment of human robot cooperation. Tianyi Wang, Ke Huo, Daniel McArthur, Ze An, David Cappeleri and Karthik Ramani from Purdue University and Muzhi Han from Tsinghua University reported on their research on autonomous robotic exploration and mapping of smart indoor environments with ultra-wideband IoT devices. Because training of intelligent systems depends upon quality data, Kelleher Guerin, Luke Tuttle and Jacob Huckaby from Ready Robotics described a common platform for semantic annotation of manufacturing data for machine learning. Finally, Adam Norton and Holly Yanco from the New England Robotics Validation and Experimentation (NERVE) Center at the University of Massachusetts Lowell described metrics and evaluation methods for assessing AI Enabled robots in manufacturing.
The symposium organizers and participants hope the highly diversified contributions from industry, academia and government to create a shared roadmap will contribute to the necessary advancement of the science, technology, and talent necessary to advance intelligent manufacturing solutions for the benefit of society. The papers, presentations and roadmap of future research are available on-line at aiinmanufacturing.wixsite.com/symposium/10-year-roadmap.
References
Deloitte, 2018 Skills Gap in Manufacturing Study. Future of manufacturing: The jobs are here, but where are the people?
Kimura, R., Reeves, M. and Whitaker, K. March 22, 2019. The New Logic of Competition: Winning in the ‘20s. BCG Henderson Institute.
Koch, M., Manuylov, I. and Smolka, M. July 2019. Robots and firms. Centre for Economic Policy Research Portal.
Leurent, H., de Boer, E. and Hernandez Diaz, D. August 2018. The Fourth Industrial Revolution and the factories of the future. World Economic Forum. www.weforum.org/agenda/2018/08/3-lessons-from-the-lighthouses-beaming-the-way-for-the-4ir/
Maybury, M. T. editor. 2004. New Directions in Question Answering. AAAI/MIT Press.
Maybury, M. T. (ed.) 2020. On-line Proceedings of the AAAI Symposium on AI and Manufacturing. aiinmanufacturing.wixsite.com/symposium.
PwC. 2016. Industry 4.0: Building the Digital Enterprise – Industrial Manufacturing Key Findings.
Schrauf, S. and Reinhard Geissbauer, R. Industry 4.0: Global Digital Operations Study. April 2018.
Participating Organizations
Government
National Institute of Standards and Technology (NIST), National Science Foundation
Industry
Cognizant Technology Solutions, FogHorn, Marshall Monroe Magic, Ready Robotics Corp, Rockwell Automation, SICK, Stanley Black & Decker, Uptake
Academia
University of Arkansas, Fayetteville State University, Hohai University, University of Massachusetts Amherst, New England Robotics Validation and Experimentation (NERVE) Center at the University of Massachusetts Lowell, North Carolina State University, University of North Carolina Charlotte, Purdue University, Stanford University, University of Tennessee Knoxville, Tsinghua University
Non-Governmental Organizations
Business-Higher Education Forum (BHEF)